Maximizing Efficiency: The Role of Image Annotation Tools for Machine Learning in Business
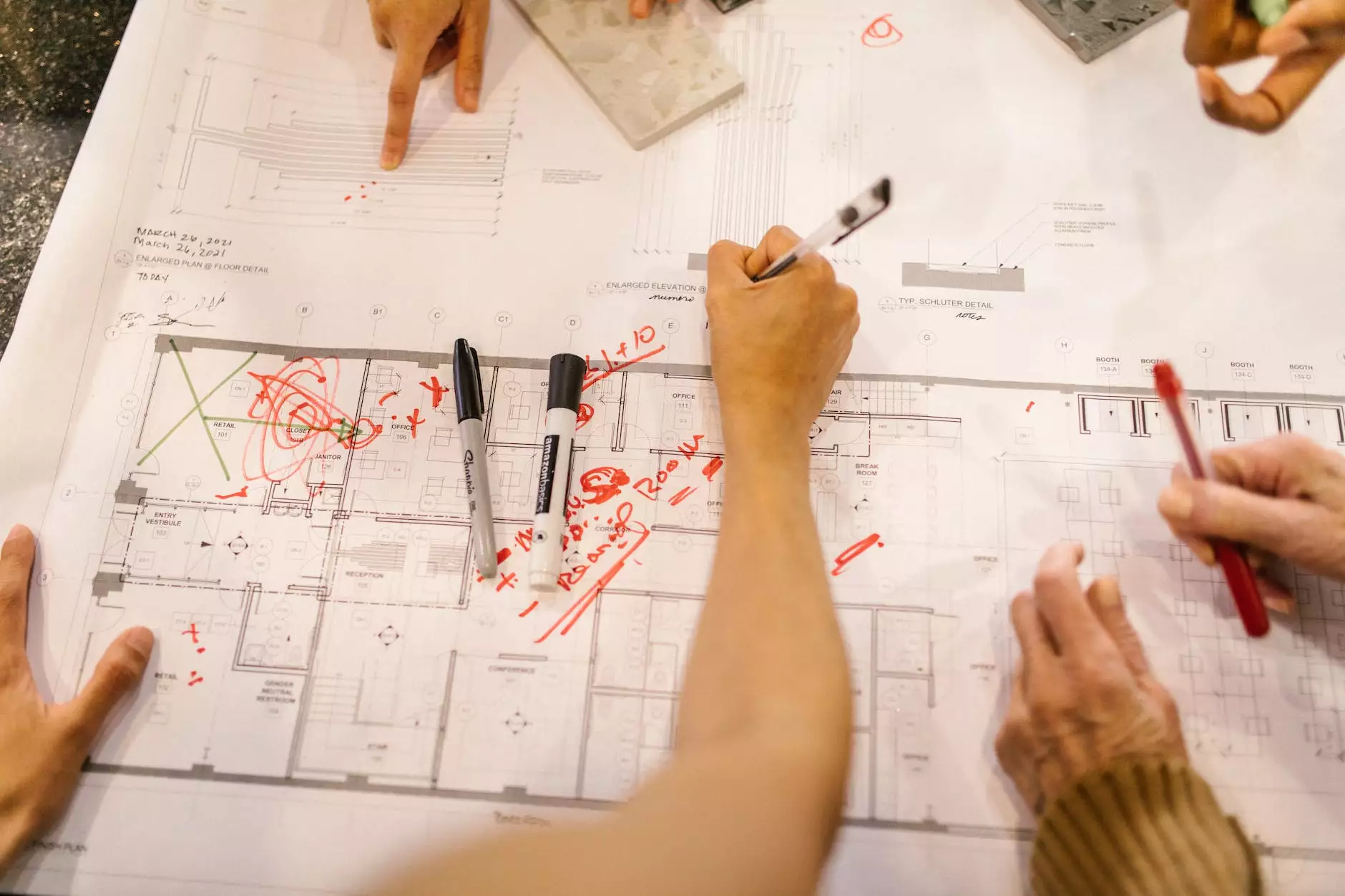
In today's fast-paced digital environment, businesses are increasingly relying on advanced technologies to gain a competitive edge. One of the most transformative aspects of this evolution is the burgeoning field of machine learning (ML), which, at its core, relies heavily on quality data input. A vital component of machine learning systems, especially those dealing with visual data, is the use of image annotation tools for machine learning.
Understanding Image Annotation in Machine Learning
Image annotation refers to the process of labeling images to provide context for machine learning algorithms. This context enables machines to recognize objects, understand scenes, and interact with their environment more effectively. With the increasing demand for automated systems capable of processing visual information, image annotation has emerged as a critical step in training machine learning models.
Importance of Image Annotation Tools
Image annotation tools serve as the bridge between raw image data and actionable insights. For businesses leveraging machine learning, understanding the significance of these tools is paramount:
- Enhancing Data Quality: Effective annotation improves the accuracy of ML models by ensuring that the data fed into these systems is relevant and correctly labeled.
- Time Efficiency: Automated annotation tools can significantly reduce the time spent labeling images, enabling teams to focus on strategic tasks.
- Cost-Effectiveness: By streamlining the annotation process, businesses can save on labor costs and allocate resources more effectively.
- Scalability: As businesses grow, so does the need for more annotated data. Scalable annotation solutions allow for faster expansion without a compromise on quality.
Choosing the Right Image Annotation Tool
With numerous options available on the market, selecting the appropriate image annotation tool for your machine learning needs can be daunting. Here are critical factors to consider:
1. Types of Annotation Supported
Different projects require different annotation types. Some common types include:
- Bounding Boxes: Used to identify and locate objects within an image.
- Semantic Segmentation: Involves classifying each pixel in an image, allowing for detailed parsing of visual content.
- Keypoint Annotation: Essential for understanding and recognizing human activities and gestures.
- Polygonal Annotation: Useful for irregularly shaped objects needing precise labeling.
2. User Interface
A user-friendly interface can greatly impact the speed and accuracy of the annotation process. Tools that feature intuitive navigation and customizable settings allow for a seamless experience.
3. Integration Capabilities
Your chosen tool should smoothly integrate with your existing workflow and systems. Look for solutions that are compatible with other software used in your operation.
4. Quality Control Features
Maintaining high-quality annotations is critical. Opt for tools that include quality assurance processes to minimize human error and reinforce data integrity.
Practical Applications of Image Annotation Tools in Business
Image annotation tools for machine learning find numerous practical applications across various sectors:
1. Retail and E-commerce
In the retail sector, image annotation can help in automating inventory tracking, analyzing customer interaction with products, and improving recommendations. For instance, retailers can leverage annotated images to train models that optimize product placement and visualize customer behavior patterns.
2. Automotive and Transportation
Self-driving technology is revolutionizing the automotive industry. Image annotation plays a critical role in training computer vision systems for vehicles, enabling them to recognize pedestrians, road signs, and obstacles through annotated visual data.
3. Healthcare
In healthcare, image annotation is vital for medical imaging analysis. Annotated medical images assist in diagnosing conditions by training algorithms to identify anomalies such as tumors and other diseases efficiently.
4. Agriculture
Farmers are adopting precision agriculture practices that utilize machine learning for crop monitoring and disease prediction. Annotated images can help train models to detect crop health and assess soil conditions.
Best Practices for Image Annotation
- Establish Clear Guidelines: Develop a set of standards and protocols to ensure consistency across annotations.
- Regular Training: Implement ongoing training for annotators to stay updated on tools and techniques.
- Use Multiple Annotators: Having multiple annotators review the same image can provide checks and balances, enhancing accuracy.
- Feedback Loop: Create a system where annotators can receive and provide feedback to continually improve the quality of annotations.
The Future of Image Annotation in Business
As businesses continue to adopt machine learning technologies, the demand for efficient and effective image annotation will only grow. Innovations in AI and automation will likely lead to more sophisticated annotation tools that further reduce manual labor and increase overall productivity.
Embracing AI-Powered Annotation Tools
AI-powered image annotation tools are emerging as game-changers in the industry. These tools utilize machine learning algorithms to automate the annotation process, providing a quicker turnaround while maintaining accuracy. Businesses that embrace AI solutions can expect to see:
- Faster Model Training: Reducing the time required for data preparation significantly speeds up the entire machine learning pipeline.
- Improved Accuracy: AI-assisted annotation can lead to more accurate results by minimizing subjective bias introduced by human annotators.
- Cost Savings: With automation, businesses can lower operational costs, freeing up resources for other essential initiatives.
Conclusion
In an era defined by digital transformation and data-driven decision-making, the importance of image annotation tools for machine learning cannot be overstated. These tools are not merely optional enhancements but essential components that can empower businesses to utilize visual data effectively, thus driving innovation and maintaining a competitive edge.
As you explore the possibilities of machine learning and its applications within your organization, consider investing in quality image annotation tools. The right tools, combined with strategic implementation, can lead to substantial improvements in productivity, efficiency, and ultimately, business success. Start leveraging image annotation tools for machine learning today and take your business operations to the next level.
image annotation tool for machine learning